Machine Learning: Clustering and Retrieval
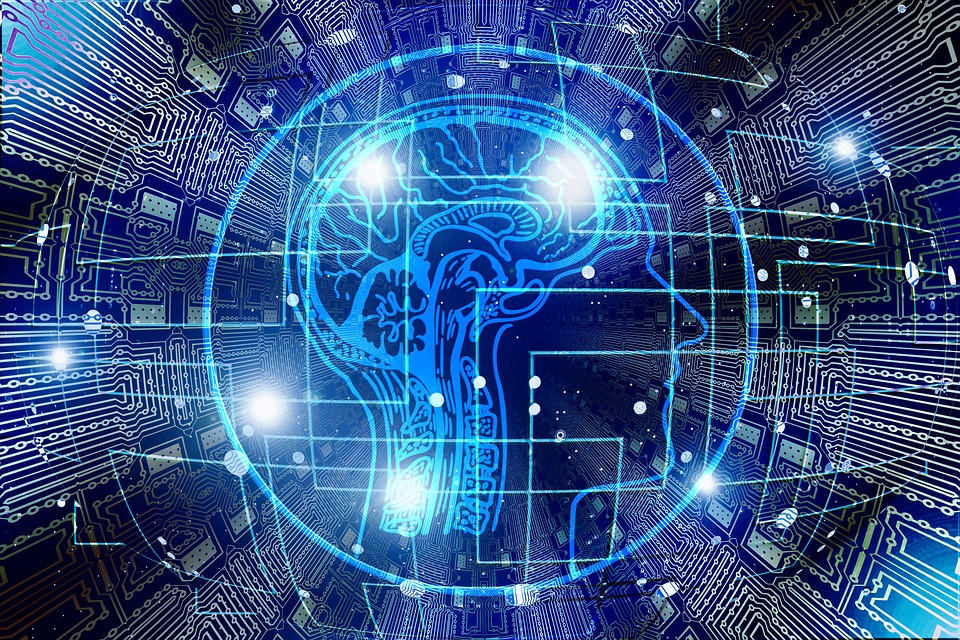
ABOUT THE COURSE!
This fourth course of the Machine Learning Program aims at providing learners with interesting topics of the most flexible and useful Machine Learning tool - Clustering and Retrieval. Unlike the previous Machine Learning processes with supervised labels and determined goals, this course will focus on extracting valuable information from seemingly unorganized and unlabeled data, which often exists in vast quantity and remained unused otherwise. While it can use data in a largely raw state (as it can not use human effort to augment the data), it is also true that we have less control over the process; thus, it is often used as either an analytic tool to aid data scientists or an auxiliary tool to help supervised processes achieve better results. The unsupervised Machine Learning is largely divided between Clustering and Retrieval. Particularly, it focuses on the use of splintering data into clustering of similar data points and detecting the important information within the data itself, and each of those problem has several approaches with different characteristics that you must acquire to best apply to a specific set of data.
To begin the course, let's take a few minutes to explore the course site. Review the material we’ll cover each week, and preview the assignments/projects/quizzes you’ll need to complete to pass the course.
Main concepts are delivered through videos, demos and hands-on exercises.
COURSE INFORMATION
Course code: | MLP304x |
Course name: | Machine Learning: Clustering and Retrieval |
Credits: | 3 |
Estimated Time: | 6 weeks. Student should allocate at average of 2 hours/a day to complete the course. |
COURSE OBJECTIVES
After taking this course, the students should all be able to:
- Understand General Idea of Clustering and Retrieval
- Understand Nearest Neighbor Search Algorithms
- Catch up idea of Kmeans Algorithm and Understand how it works
- Understand Mixture Models Idea
- Understand a combined way between Mixed Membership Modelling and Lattent Dirichlet Allocation
- Understand another approach in the Clustering problem, the purpose of this lesson is to make more ways to perform the clustering problem
- Do assignment to make clear about clustering problem deeply.
COURSE STRUCTURE
Module 1 - Fundamental Clustering algorithms
- Lesson 1 - Introduce to Clustering and Retrieval tasks
- Lesson 2 - Introduction to nearest neighbor search and algorithms
- Lesson 3 - The importance of data representations and distance metrics
- Lesson 4 - Scaling up k-NN search using KD-trees
- Lesson 5 - Locality sensitive hashing for approximate NN search
Module 2 - Clustering with k-means
- Lesson 6 - Introduction to clustering
- Lesson 7 - Clustering via k-means
- Lesson 8 - MapReduce for scaling k-means
Assignment 1 - Project - Building a movie recommendation system
Module 3 - Mixture Models
- Lesson 9 - Motivating and setting the foundation for mixture models
- Lesson 10 - Mixtures of Gaussians for clustering
- Lesson 11 - Expectation Maximization (EM) building blocks
- Lesson 12 - The EM algorithm
Module 4 - Mixed Membership Modeling via Latent Dirichlet Allocation
- Lesson 13 - Introduction to latent Dirichlet allocation
- Lesson 14 - Bayesian inference via Gibbs sampling
- Lesson 15 - Collapsed Gibbs sampling for LDA
- Lesson 16 - Hierarchical clustering and clustering for time series segmentation
- Lesson 15 - Collapsed Gibbs sampling for LDA
Assignment 2 - Project - Augment Classification by Topic Modeling
DEVELOPMENT TEAM
COURSE DESIGNERS
Ph.D. Nguyen Van Vinh |
|
B.A. Nguyen Hoang Quan |
|
|
|
REVIEWERS & TESTER
Course Reviewer |
Course Tester |
||
Ph.D. Tran Tuan Anh |
|
M.Sc. Nguyen Hai Nam |
|
Program Reviewers
|
||
Assoc. Prof. Tu Minh Phuong Dean of IT Faculty Posts and Telecommunications Institute of Technology (PTIT) |
Ph.D. Hoang Anh Minh R&D Manager, FPT Software Chief Scientist, LA Office |
Ph.D. Le Hai Son Machine Learning Expert FPT Technology Innovation |
MOOC MATERIALS
Below is the list of all free massive open online learning sources (MOOC) from Coursera used for this course by FUNiX:
Learning resources
In modern times, each subject has numerous relevant studying materials including printed and online books. FUNiX Way does not provide a specific learning resource but offers recommendation for students to choose the most appropriate source to them. In the process of studying from many different sources based on that personal choice, students will be timely connected to a mentor to respond to their questions. All the assessments including multiple choice questions, exercises, projects and oral exams are designed, developed and conducted by FUNiX.
Learners are under no obligation to choose a fixed learning material. They are encouraged to actively find and study from any appropriate sources including printed textbooks, MOOCs or websites. Students are on their own responsibilities in using these learning sources and ensuring full compliance with the source owners’ policies; except for the case in which they have an official cooperation with FUNiX. For further support, feel free to contact FUNiX Academic Department for detailed instructions.
Learning resources are recommended below. It should be noted that listing these learning sources does not necessarily imply that FUNiX has an official partnership with the source’s owner: Coursera, tutorialspoint, edX Training, Udemy or Standford.
Feedback channel
FUNiX is ready to receive and discuss all comments and feedback related to learning materials via email program@funix.edu.vn